Research powered by XNAT: TRACERX-RENAL
XNAT is an advanced management system for research images. It uses multiple technologies to provide researchers with the tools they need to process data and view data. The ICR has been at the forefront of developing new capabilities for XNAT, in particular, the ICR-XNAT-OHIF viewer (see Doran et al. Tomography 8.1 (2022). The XNAT Team has the privilege of working with outstanding scientists both locally and internationally, and our tools help them to deliver high-impact clinical studies. This page describes a collaboration between the Institute of Cancer Research and The Francis Crick Institute.
Matthew R Orton, Evan Hann, Simon J Doran, Scott TC Shepherd, Derfel Ap Dafydd, Charlotte E Spencer, Jose I Lopez, Víctor Artahona, Francesca Castagnoli, Hannah Warren, Joshua Shur, Christina Messiou, James Larkin, Samra Turajlic on behalf of the TRACERx Renal Consortium, Dow-Mu Koh. Interpretability of radiomics models is improved when using feature group selection strategies for predicting molecular and clinical targets in clear-cell renal cell cancer: insights from the TRACERx Renal study Cancer Imaging 23(1), 76 (2023)
Xiao Fu, Yue Zhao, Jose Lopez, Andrew Rowan, Lewis Au, Annika Fendler, Steve Hazell, Hang Xu, Stuart Horswell, Scott Shepherd, Lavinia Spain, Fiona Byrne, Gordon Stamp, Tim O’Brien, David Nicol, Marcellus Augustine, Ashish Chandra, Sarah Rudman, Antonia Toncheva, Andrew Furness, Lisa Pickering, Matthew Orton, Simon Doran, Dow-Mu Koh, Christina Messiou, Derfel ap Dafydd, Santosh Kumar, James Larkin, Charles Swanton, Erik Sahai, Kevin Litchfield, Samra Turajlic, Paul Bates, Spatial patterns of tumour growth impact clonal diversification: computational modelling and evidence in the TRACERx Renal study, Nature Ecology and Evolution, 6(1), 88–102(2022)
FEATURED PUBLICATIONS: TRACERX-Renal study
The ICR has developed a highly successful partnership with the Francis Crick Institute, which has recently resulted in two high-impact publications. TRACERx Renal (NCT03226886) is an ongoing prospective multi-omics study exploring the genomic and molecular drivers of clear-cell renal cell carcinoma (ccRCC) with a target accrual of 320 patients.
Xiao et al. Nature Ecology and Evolution (2022) creates an in silico model of tumour growth and demonstrates how specific patterns of proliferation and necrosis explain clonal expansion and emergence of parallel evolution and microdiversity in tumours. Simulated time-course studies reveal the appearance of budding structures before detectable subclonal diversification. Intriguingly, regions-of-interest annotated in XNAT show radiological evidence of budding structures in early-stage clear cell renal cell carcinoma, indicating that future clonal evolution may be predictable from imaging.
The interim results of the first 101 patients provided novel insights into cancer evolution and identified novel prognostic genetic features (Turajlic S, et al. Cell 173(3):595-610.e11 (2018)). Specifically, patient outcome could be independently predicted from weighted genomic instability index (wGII, a measure of chromosomal complexity), and genetic intratumoural heterogeneity (ITH, a reflection of diversity of the genetic landscape of the tumour cells). Directly translating such biomarkers into the clinic is currently not possible since they necessitate cost-prohibitive genomic profiling. Furthermore multiple tumour samples are required to accurately capture ITH, which is only possible from post-surgical resection of the tumour. Thus, these biomarkers cannot currently be applied clinically for treatment planning or determining surveillance regimens in patients who do not have surgery. A method that could classisfy patients solely on the basis of non-invasive pre-surgical imaging could have important implications.
XNAT was chosen to manage and manipulate image data because of the ability to annotate images with machine-readable regions of interest. Scan data were anonymised by the XNAT Team and an XNAT-enabled image preprocessing workflow was created to re-sample the data to a consistent resolution. Segmentations on multiple slices were created using the ICR-XNAT-OHIF viewer. Two different image sub-segmentation methods were explored: sub-segmentation into visually apparent high and low
enhancing sub-regions, and erosion of the tumour masks
by 10mm to generate core and rim masks.
The study led to the development of two novel feature-selection strategies for use with radiomics studies and demonstrated that their combined use yields models with equivalent performance to a widely used pipeline. The proposed strategies also lead to models with fewer features meaning model interpretation is more straightforward across a range of eleven molecular, histopathological and clinical targets.
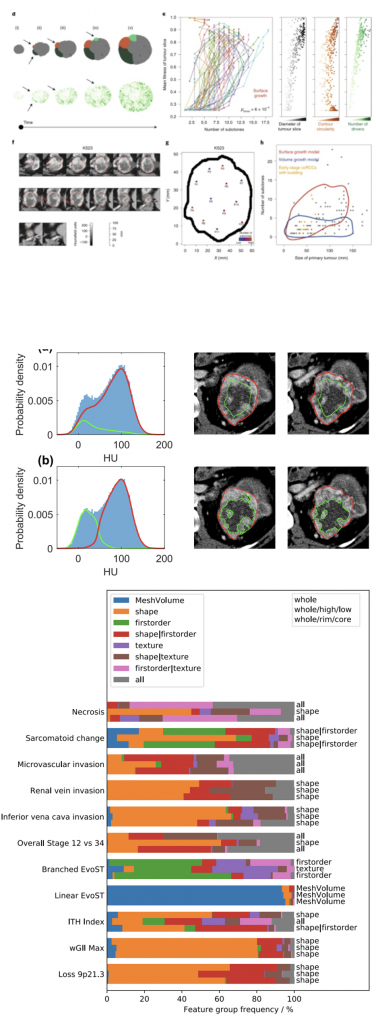