Multicentre trials powered by XNAT for RM
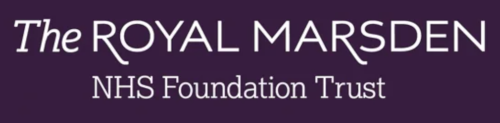
XNAT is an advanced management system for research images. It uses multiple technologies to provide researchers with the tools they need to process data and view data. The RM has been running large multicentre imaging trials using XNAT since 2020.
List of studies using XNAT in collaboration with the Royal Marsden
[1] RDC-BIO: Rapid Diagnostic Centre Biomarker Study. NCT05876676. Project Lead: Dr Richard Lee (Royal Marsden). Rapid Diagnostic Centres(RDC) were built to diagnose patients who have common symptoms that occur in cancer, but it is unclear if they have cancer or not, i.e., non-specific but concerning symptoms (NSCS). These symptoms include: weight loss, fatigue, cough, GP suspicion. Only 1 in every 10 patients (10%) referred to an RDC will have cancer. Some of the patients with cancer may have been more likely to develop cancer due to inherited or environmental factors. Some of the patients who don’t have cancer may also be at higher risk of developing cancer at another time due to inherited or environmental factors.
[2] FAIR-O: Feasibility of Frailty Assessment and Implementation of Interventions in Women Over 70 With Epithelial Ovarian Cancer. NCT04300699. Project Lead: Prof Susana Bannerji (Royal Marsden). This study aims to assess whether oncology teams can deliver a geriatric assessment and targeted interventions to address issues identified according to the study algorithms. The study algorithms have been designed by a multidisciplinary team including consultant geriatricians with specialist interest in geriatric oncology. The secondary objective of the trial is to determine whether sarcopenia and reduced muscle attenuation at baseline, predicts for reduced tolerance to chemotherapy, functional decline, and poorer survival outcomes; to assess whether loss of muscle mass during chemotherapy is associated with reduced tolerance to chemotherapy and functional decline; to explore the relationship between frailty, as identified in a comprehensive geriatric assessment, and sarcopenia. A particular driver for using XNAT in this study is its ability to deploy an AI algorithm, developed by collaborators at Imperial College, which can automatically segment muscle and thus quantitatively measure the sarcopenia-related loss in muscle mass.
[3] NIMBLE: Nodule IMmunophenotyping Biomarker for Lung Cancer Early Diagnosis Study. NCT05432739. Project Lead: Dr Richard Lee (Royal Marsden). NIMBLE is a prospective study for blood biomarker study of lung nodules alongside analysing data which has been collected routinely as part of patient care. The primary aim of NIMBLE is to assess whether artificial intelligence and machine learning based radiomics approaches can be used to distinguish between benign disease and malignancy in a new lung nodule after a previously treated cancer, and where malignant to differentiate between metastatic recurrence or a new primary lung cancer.
[4] AI-SONAR: AI & Radiomics for Stratification of Lung Nodules After Radically Treated Cancer. NCT05375591. Project Lead: Dr Richard Lee (Royal Marsden). This retrospective study is assessing the utility of radiomics and artificial intelligence approaches to new lung nodules in patients who have undergone radical treatment for a previous cancer. It entails use of machine learning (ML) approaches and later, exploration of deep-learning/convolutional neural network approaches to nodule interpretation for differentiation of benign, metastatic and new primary lung cancer nodules/lesions. Development of a ML classifier or deep learning based tool may help guide which patients would benefit from earlier investigations including additional imaging, biopsy sampling and lead to earlier cancer diagnosis, leading to better patient outcomes in this unique cohort.
[5] MALIMAR: MAchine Learning In MyelomA Response. NCT03574454. Project Lead: Prof Andrea Rockall (Imperial College London). MALIMAR is an observational clinical study combining “real world” and clinical trial data, both retrospective and prospective. Radiological reporting of myeloma requires inspection over the whole imaging volume of at least 7 different image series: T1-weighted Dixon “in-phase”, “out-of-phase”, “fat” and “water”; two DWI “b-values”; and an apparent diffusion coefficient (ADC) map. Patterns of marrow infiltration, burden of disease and degree of response all influence prognosis, but objective quantification is challenging, in principle requiring the generation of large numbers of regions-of-interest (ROIs) in 3-D on multiple image contrasts and the derivation of quantitative imaging biomarkers. Manual analysis of this nature is impractical in the clinical workflow, and this is an area that is ripe for the use of artificial intelligence (AI). However, the creation of robust and generalisable automated analysis tools first requires the assembly of large and sufficiently diverse datasets to support model training and validation. XNAT was perfect to achieve this ambitious goal – see our featured publication.
[6] OCTAPUS-AI: Optimising Cancer Therapy And Identifying Causes of Pneumonitis USing Artificial Intelligence (COVID-19). NCT04721444. Project Lead: Dr Richard Lee (Royal Marsden). This completed study aimed to develop and validate a machine learning classifier to distinguish between COVID-19 and cancer therapy (radiotherapy and immunotherapy) induced lung changes and to develop a prognostic machine learning signature for recurrence of non-small cell lung cancer following radical radiotherapy to stratify appropriate surveillance and onward care, thus minimising unnecessary hospital visits and resource use. Data collected have allowed identification of lung pathology in cancer patients related to therapy or COVID-19 induced pneumonitis and the prognostication and stratification of follow-up in NSCLC patients treated with radical thoracic radiotherapy in the COVID-19 era. See our featured publication.
[7] DISCOVAR: Diffusion-weighted Imaging Study in Cancer of the Ovary. NCT01505829. Project Lead: Prof Nandita deSouza (Royal Marsden and ICR). This completed study sought to develop measurement of diffusion as a quantitative imaging biomarker for evaluating and monitoring treatment response in ovarian cancer metastases and to assess its potential in monitoring treatment response. The study involved standardising DW-MRI for the abdomen and pelvis across multiple centres and platforms, assessing reproducibility of the measurement in patients planned for neoadjuvant chemotherapy and assessing its utility as an early response biomarker in patients with platinum-sensitive relapse due to receive therapy with carboplatin. Scanning measurements were correlated with histopathological markers in tumour samples in order to link the biomarker with response mechanisms.